Hacking Democracy Conference
How Can Technology Help to Facilitate Transitional Justice?
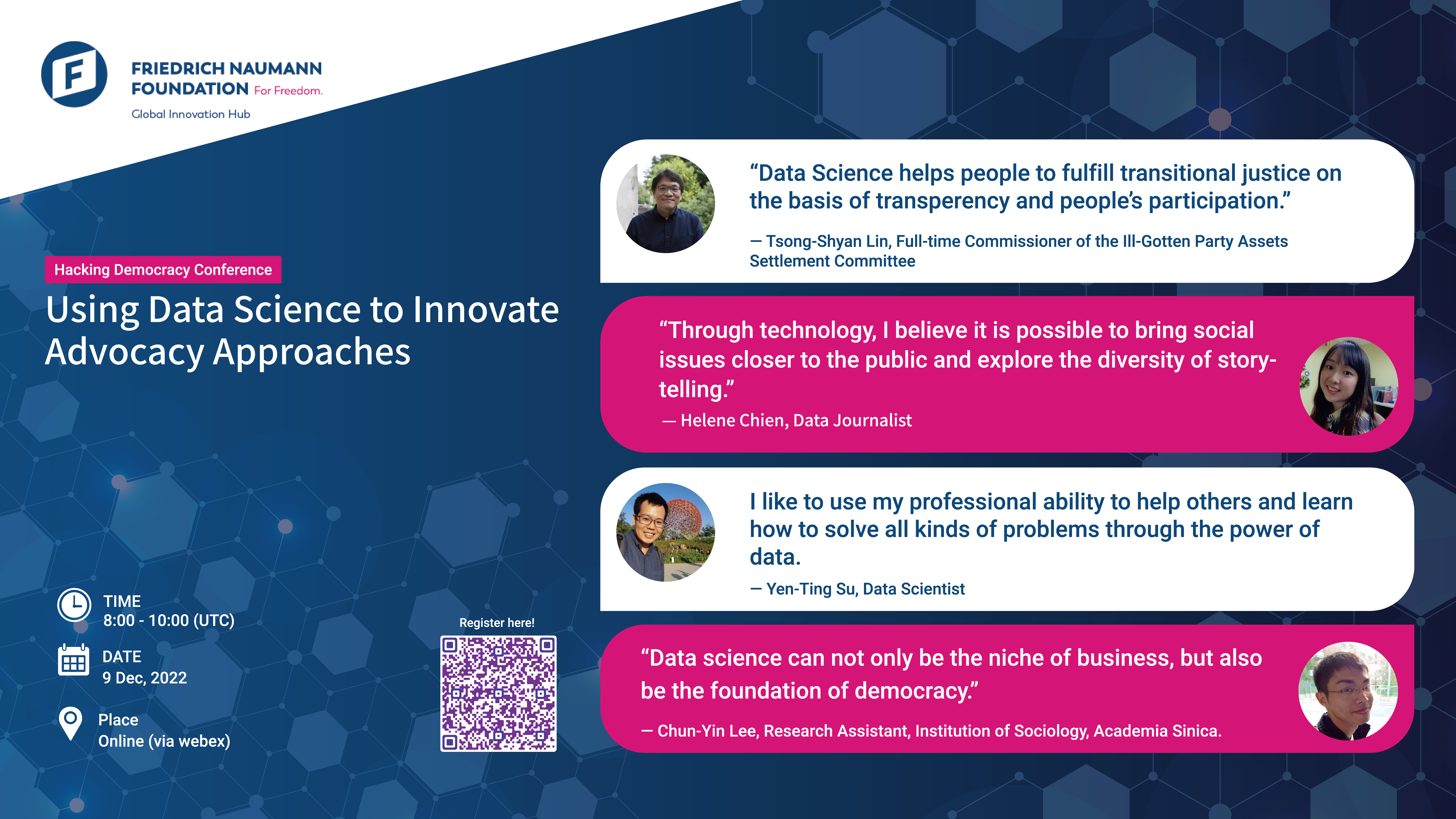
Before FNF’s Hacking Democracy Conference on December 9, let’s learn how the Conference’s panelists built the Textual Analysis System and Digital Narratives Project for Ill-gotten Party Assets Settlement Committee (CIAS). Click here to register for the Conference to learn more!
The Textual Analysis System and Digital Narratives project for Taiwan’s Ill-gotten Party Assets Settlement Committee (CIPAS) originated in the Data for Social Good (D4SG) Fellowship. The Fellowship was initiated by DSP Inc. in line with the idea of using data power for the public good. A data science team consisting of volunteers from various fields uses data to solve problems facing non-profit organizations and the public sector.
The Ill-gotten Party Assets Settlement Committee (CIPAS) was established in 2016 by Taiwan’s government to achieve transitional justice. CIPAS’s purpose is to investigate whether any political party still possess any ill-gotten assets which the party acquired by its authoritarian rule during Taiwan’s marital law period. Particularly, many of these assets are actually national property and thus should be returned to the people and the government.
Team members of this project come from academia, industry, and the media. Together with the Ill-gotten Party Assets Settlement Committee (CIPAS), we have proposed setting up a visualized textual analysis system for data exploration and producing digital special reports to optimize the reading experience. This project gathers dispersed and complex cultural and historical materials and presents them in systematic fashion to enable researchers to explore history more efficiently. When a researcher completes a project investigation report, we transform it into an interactive digital report that will engage readers, in order to enhance public understanding of and interest in transitional justice.
Key problems: Historical materials are scattered and complex, investigative reports have limited reach
CIPAS was set up in 2016 as a task force under the Executive Yuan (Cabinet). Its goal is to implement transitional justice by investigating and dealing with the improperly acquired property of political parties, affiliated organizations, and their trustees; establishing a fair competitive environment for political parties; and improving democratic politics. To this end, CIPAS collected thousands of pages of historical materials, digitized some by hand, had them analysed and interpreted by researchers, and published investigative reports.
Researchers have to spend a lot of time reading historical materials and looking for connections in the sea of scattered historical materials in order to clarify the relationships between people and organizations within a given context. However, researchers specialize in different areas: so, although each researcher has a deep, thorough understanding of their own domain, extensive knowledge and experience are required if they are to construct a complete picture. Finding links between the historical materials in various fields will help us to carry out in-depth and comprehensive descriptions and analyses of the history of the previous one-party state era in Taiwan.
Although CIPAS regularly publishes investigative reports and historical accounts, it is constrained by its own rigorous requirements on formatting and wording, and by the need to quote a large amount of difficult historical materials; in addition, the texts contain a vast amount of professional terminology. This makes it difficult for members of the public to absorb information or understand the achievements and goals of CIPAS, and may even cause misunderstanding.
To resolve these problems, we have outlined two major directions for this collaborative project:
-
To reduce research time that researchers need to spend for exploring historical documents by decreasing the amount to be read and interconnected, even allowing researchers to use simple data science tools to explore relationships between historical figures and the historical context of events relating to a specific theme.
- To convert rigorous, lengthy reports into forms that are easier for people to understand, and to optimize the reading experience for ordinary people.
Creating an optimized search system to support internal users: Word segmentation, named entity recognition, social network analysis, and article recommendation system
To build a systematic historical data analytical tool, we first drew on 298 electronic historical records released on the CIPAS website and used the word segmentation system developed by the Chinese Knowledge and Information Processing (CKIP) team at Academia Sinica. The system’s word segmentation and named entity recognition technologies look at the words in a text and determine their parts of speech, while also identifying names of people and organizations.
In English, each word is divided by a blank. On the other hand, languages such as Chinese and Japanese, a word may consist of one, two, or even four characters, and each word in a sentence is put together instead of being divided by a blank. Therefore, if people want to find and analyse words in an article in such languages, they have to apply word segmentation program to quick divide each word in articles.
It should be noted that the CKIP system was developed for general application, so lexical analysis for specific fields requires support from experts in those fields, which required us to construct a dictionary of proper nouns relating to ill-gotten party assets. In addition, the word segmentation system cannot pre-integrate synonyms, so a single person may appear under various names in CIPAS historical materials (e.g. “Chiang Kai-shek”, “Chiang Chung-cheng”, and “Generalissimo Chiang” all refer to the same person). Thus, we worked with researchers to build a specialist CIPAS lexicon and define synonyms so as to increase the accuracy of word segmentation. We also designed an “Add Words” function to enable researchers to easily add words in the future.
Next, we constructed a word vector matrix and calculated the degree of correlation between articles. The more similar the vocabularies used by two different articles, the higher the correlation between those articles, thus giving rise to an article recommendation system.
We also used social network analysis (SNA) to show the association of people or institutions under specific topics. Social network analysis is an analytical technique that focuses on relationships but is also a data visualization tool. We used the CIPAS lexicon described above to create a list of people and organizations and sort through items occurring on this list in the article database. Where items appear together in the same article, they are considered to be related or linked, thus yielding a social network map. Below is a diagram of the institutional network in the “National Women’s League of the Republic of China (NWL)” article database.
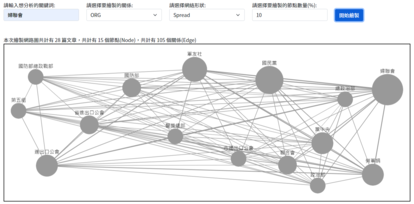
Figure 1. An example of how social network analysis was applied in this project.
© Yen-Ting Su (蘇彥庭) and Chun-Yin Lee (李俊穎) are the authors of this picture and have authorized FNF to use it following CC BY-NC-ND 4.0.The two important elements in the network diagram are nodes and edges. Edges means the lines connecting each node on the picture. A node represents a person or an organization, and an edge connects two nodes that appear together in the article. We constructed the nodes such that their size reflects the importance of the people or organizations they represent in the subject article database. Meanwhile, the strength of a connection is represented by the thickness of the edge: the higher the number of co-occurring articles, the thicker the line. These techniques add informational richness to the data visualization process. Finally, in order to effectively combine network analysis with the article database, we also provided a list of articles corresponding to nodes and edges, so that researchers can easily find related articles as they explore network relationships.
Building a friendly, concise knowledge window for the general public: Graphics, data visualization, interactive charts, and webpage templates
Many reports are tens of thousands of words long and use difficult, technical vocabulary. Thus, to optimize the reading experience, our intervention takes two forms: textual content and webpage function.
Textual content must be focused around a specific theme, and the weight of information must be controlled in order to avoid digression. Once the subject matter is decided, it is necessary to summarize and interpret the information, extract important data and interesting accounts, and convert technical terms (legal, accounting, political etc.) into everyday language.
In addition, human attention tends to be vision-centred. We found that a lot of unique audiovisual and graphic materials can be found in historical materials and in hearings that CIPAS held as a due process to investigate every ill-gotten party assets case by inviting all the individuals or organizations involved. Processing and reusing these materials can also add the finishing touch to the report that were mostly presented though texts, numbers, and pictures.
Data visualization is very important to webpage function. CIPAS investigative reports take stock of the property and land data of many institutions. We converted these data into maps or interactive statistical charts, so that readers can see the data stories at a glance and can also explore individual records one by one. Presenting the information with a navigation bar and a menu also allows readers to get an overview of the general content and control their reading progress, which reduces the risk of their becoming impatient or distracted.
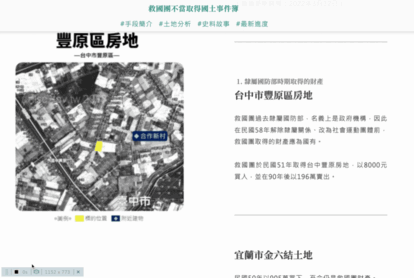
Figure 2. This picture demonstrated how digital narrative was applied in this project.
© Helene Chien (簡毅慧) is the author of this picture and has authorized FNF to use it following CC BY-NC-ND 4.0. The materials of this pictures were made or provided by Ill-gotten Party Assets Settlement Committee. If you would like to use it, please follow its license statement. This report which this picture demonstrated will be published on https://www.stories.cipas.gov.tw/.Coda
Historical materials can often be dull or uninteresting. Everything that CIPAS researchers discovered from the vast stream of historical materials could be a key to find the truth behind the previous authoritarian history and thus illuminate the path to advance democracy. However, transitional justice is not simply a matter untangling the truth from a complicated history. It must also use appropriate methods if it is to be accessible and understandable to the public. Only in this way can we build consensus and realize transitional justice.
This project came about in part thanks to DSP Inc. for finding “data heroes and heroines” who are willing to contribute their skills or experience, but also because a group of social actors are pursuing transitional justice and daring to try a variety of methods, integrating CKIP’s word segmentation and named entity recognition technologies as well as social network analysis to identify correlations between historical materials in graphic form.
When faced with a large number of historical documents, such analytical methods can help researchers comb through materials more efficiently. At the same time, once researchers have written their reports, they can use digital editorials to promote their findings and thus make it easier for the public to access information on transitional justice issues.
In summary, we hope the technologies developed with current data science can provide people fighting for transitional justice in Taiwan and around the world with better tools for use in their research and activism.
Where to learn more?
For further information on this project, click on the links below:
* Internal tool: Optimized search system
* Digital editorial: China Youth Corps property
*This article was jointly written by Mr. Tsong-Shyan Lin (Full-time Commissioner of the Ill-Gotten Party Assets Settlement Committee), Ms. Helene Chien (Data Journalist), Mr. Chun-Yin Lee (Research Assistant of Institution of Sociology, Academia Sinica), and Mr. Yen-Ting Su (Data Scientist).